Machine learning to develop safer batteries
Marika Yang
Dec 20, 2018
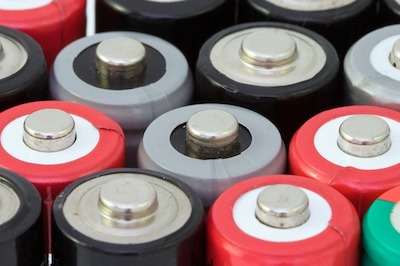
Source: Pixabay
Solid electrolytes hold promise for the development of safer batteries, but selecting the best materials could require years of analysis. Can machine learning speed up the process?
Electronics are essential to everyday life. What would our lives be like without our cell phones or computers? From toys to laundry machines to electric cars, electronics continue to populate our daily routines. Many of these electronics are powered by high energy density lithium-ion batteries. But two factors in these batteries can lead to dangerous consequences.
First, the battery electrolyte, the material that conducts ions between the cathode and the anode, is typically a liquid, which is flammable. Second, as batteries are charged and discharged over time, uneven lithium deposition at the anode can lead to the growth of dendrites which can connect the cathode and anode. This can lead to fires and explosions because of the electrolyte’s flammability. Both Tesla cars and Boeing aircraft have suffered from dendrite battery problems, and sudden cell phone explosions can also be attributed to dendrites.
This is the problem that Zeeshan Ahmad and Tian Xie are trying to solve. Ahmad, a Ph.D. candidate in mechanical engineering at Carnegie Mellon University, and Xie, a Ph.D. candidate in materials science and engineering at the Massachusetts Institute of Technology, recently published a paper about their research to find possible solutions to this dendrite problem. They turned to machine learning to generate and analyze massive amounts of data to find these solutions.
We had 13,000 inorganic crystalline materials to screen over for the solid electrolyte... we used machine learning because it can work on large data scales.
Zeeshan Ahmad, Ph.D. student, Mechanical Engineering
To suppress the growth of dendrites, Ahmad and his colleagues researched potential solid electrolytes, which unlike liquid electrolytes, are not flammable. Not only did they decipher what properties the solid electrolyte requires, they also needed to analyze thousands of possible solid materials, which would have taken years using traditional experimental methods.
“We had 13,000 inorganic crystalline materials to screen over for the solid electrolyte,” Ahmad said. “It was hard to just calculate the properties of each solid electrolyte separately because it’s very computationally expensive. We used machine learning because it can work on large data scales, to find out the properties of solid electrolytes.”
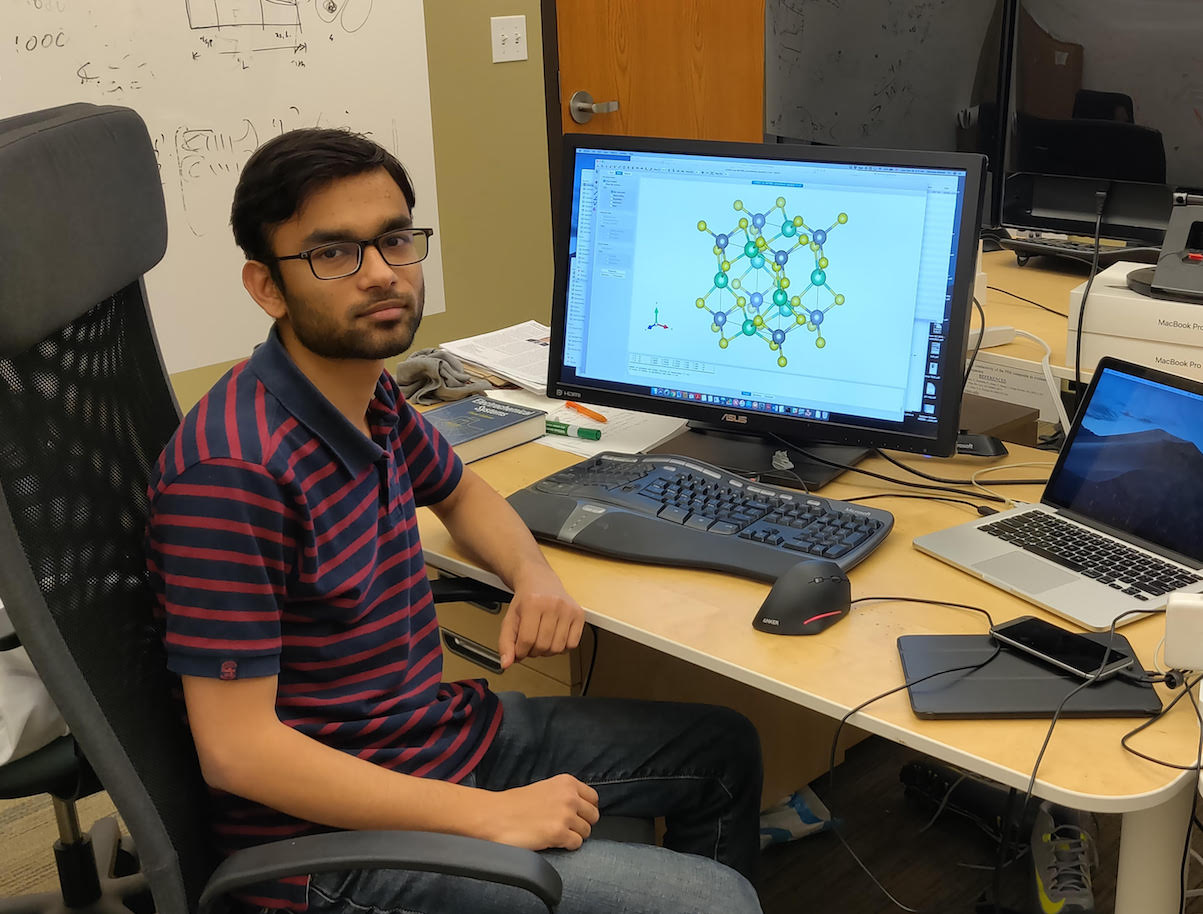
Source: College of Engineering
Zeeshan Ahmad in the lab.
Due to the range of data availability, Ahmad and Xie used a variety of machine learning models. For cases with sufficient existing data that can be used for training the model, they used a graph convolutional neural network model to predict the properties of inorganic crystalline solid electrolytes. In cases where there wasn’t sufficient training data, they used linear regression with regularization, which is more suitable for low data problems.
“We tested all these materials for design criteria or solid electrolytes,” Ahmad said. “Our solid electrolytes should suppress dendrite growth at the lithium metal anode and be electronically insulating. They should be stable—they should not decompose spontaneously at room temperature. They should conduct ions very fast to achieve a high power density of the battery required for fast charging.”
After analyzing the solids, they found six possible materials that could be used as solid electrolytes, belonging to the sulfide, iodide, and borohydride classes.
“We are very excited to apply our machine learning framework to important materials problems, which is why the collaboration with the CMU team has been so rewarding,” Xie said. “Finding six potential solid electrolytes in such a short time shows the possibility of accelerating material discovery drastically with machine learning tools.”
Now, Ahmad is working on circumventing the fundamental tradeoff between solids and liquids: liquids generally have high ionic conductivity, and very few solids that have a comparable level of conductivity are also stable enough to be used as electrolytes. Ahmad is researching the potential of composite electrolytes, combining multiple solids with different desired properties.
“We are looking into composite electrolytes, but the problem is that generally we do not have sufficient data to use machine learning,” Ahmad said. “So we are trying to address this problem through first principles methods—we use density functional theory and molecular dynamics to predict the properties. Once we do it for, let’s say, five materials, we can find out certain design principles, and then we can try to use those design principles to help us screen through other composites.”
The paper, Machine Learning Enabled Computational Screening of Inorganic Solid Electrolytes for Suppression of Dendrite Formation in Lithium Metal Anodes, was published in the journal ACS Central Science, DOI: 10.1021/acscentsci.8b00229. It was named a top ionizing paper in Research Interfaces. Additional authors were Venkat Viswanathan, an assistant professor of mechanical engineering at Carnegie Mellon, Jeffery C. Grossman, a professor of materials science and engineering at MIT, and Chinmay Maheshwari, a student at the Indian Institute of Technology, Bombay, who was a summer research intern with Viswanathan.
Media contact:
Lisa Kulick
lkulick@andrew.cmu.edu