Zhang and Yao earn MFI award for 4D printing
Researchers collaborate to develop self-assembling structures to lower manufacturing costs
Kathryn Anne Quelle
Oct 9, 2018
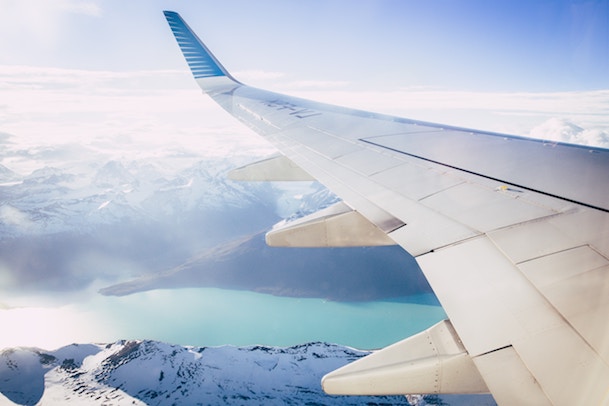
Source: Margo Brodowicz, Unsplash
Fiber-reinforced composites are used to make structural components of airplanes and cars, but they are labor-intensive to produce.
Jessica Zhang, a professor of mechanical engineering with a courtesy appointment in biomedical engineering at Carnegie Mellon University, and Lining Yao, an assistant professor of the Human-Computer Interaction Institute (HCII) with courtesy appointments in mechanical engineering and materials science and engineering at Carnegie Mellon, received a Manufacturing Futures Initiative (MFI) award for their collaborative project, “SimuLearn: Combining Machine Learning, Mechanical and Geometrical Simulation for the Inverse Design and Manufacture of Self-assembling Fiber-reinforced Composites.”
Fiber-reinforced composites (FRCs) are often used to make shells and structural components of ships, airplanes, and cars, but they are very expensive to produce. Currently, industries produce FRCs through a labor-intensive process of laminating layers of resin and fibers on top of molds. Zhang and Yao propose to work on a different method: using 3D printers to print initially flat FRC structures that can self-assemble into targeted 3D shapes when residual stresses are released – a process called 4D printing.
The project will focus on using one composite material: thermoplastic FRC, which is a thermoplastic with fiber reinforcements embedded within its matrix. The self-assembly process occurs by inducing residual stresses in the thermoplastic matrix by manipulating orientations of the fiber alignment during printing. Industries have yet to embrace printing self-assembly FRC structures because they lack the effective simulation tools needed to ensure the accurate self-assembly of complex shapes. With their project, Zhang and Yao hope to make 4D printed self-morphing materials more practical.
By merging machine learning, geometrical computation and finite element analysis, we can characterize morphing matters more accurately and efficiently.
Professors Jessica Zhang and Lining Yao, Carnegie Mellon University
The most common material transformation simulations use either geometrical computation or Finite Element Analysis (FEA). Geometric methods simulate transformations quickly but cannot capture complex designs or factor in real-world considerations, such as gravity. FEA can consider more transformation factors, but it is time-consuming and requires a lot of computation.
Zhang and Yao will specifically address the dilemma between computational cost and accuracy by incorporating machine learning into FEA simulations. They will begin by modelling their chosen composite material, thermoplastic FRC, and will proceed by running FEA transformation simulations. They will convert transformation data collected during the simulations into numeric values to train machine learning models. Zhang and Yao say, “By merging machine learning, geometrical computation and finite element analysis, we can characterize morphing matters more accurately and efficiently.”
The project will culminate in fabrication demos, where Zhang and Yao plan to demonstrate their research using a self-assembling FRC mesh and grid to make navy structures, car seats, engine covers, and helmets. Their overall project will benefit FRC manufacturers as well as computer-aided design (CAD) software companies, which will be able to improve software performance using the project’s rapid simulation tools.
Carnegie Mellon University’s Manufacturing Futures Initiative (MFI) awards fund multidisciplinary, collaborative projects of two or more faculty members. MFI awards specifically support research incorporating machine intelligence into manufacturing environments.
Media contacts:
Lisa Kulick, College of Engineering
lkulick@andrew.cmu.edu
Byron Spice, School of Computer Science
bspice@cs.cmu.edu